Artificial intelligence bank: the future of finance?
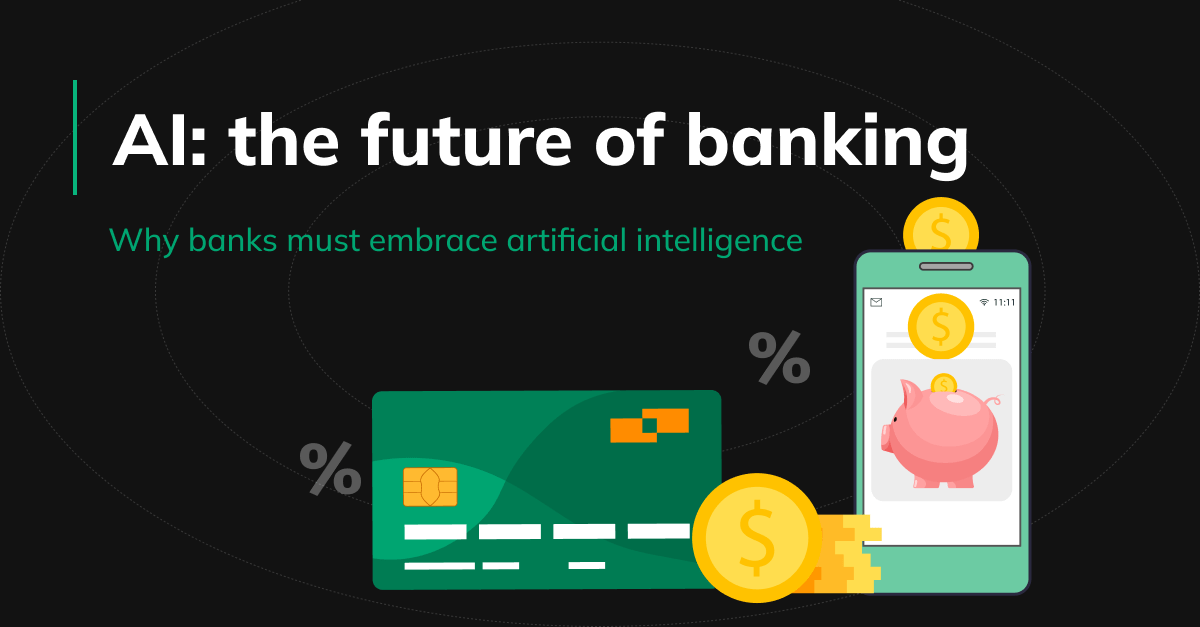
As in many other fields, artificial intelligence technologies offer a multitude of uses in the banking industry. From virtual assistants and fraud detection to predictive analytics and risk assessment backed by machine learning – AI solutions are multiple.
According to insights by McKinsey, becoming an AI-first bank is crucial for such financial institutions to stay ahead of the curve and attract clients.
If you’re a business founder with a banking app developed (or thinking about building one) and want to learn more about the potential benefits and challenges of doing so – this article is for you.
Why is it so important for banks to become “AI-first”?
Don’t get out-competed by other banks
More than half of the financial companies surveyed by McKinsey’s Global AI Survey have included at least one AI tool or feature in their services (source).
Statista predictions indicate that by the year 2030, the adoption of AI in the banking sector will generate approximately 99 billion US dollars worth of value in the Asia Pacific region alone. Missing out on AI technologies means getting out-competed by other players in the finance sector (source).
Don’t get out-competed by nonbanking businesses
Meta, Microsoft, Amazon, Google, and Apple are racing against each other to create the Western supper app – an all-in-one app that bundles services and repeats the success of the Chinese super app, WeChat.
They aim to combine all aspects of consumers’ online lives, from discovery (search, social media) to delivery. Most importantly, the Big Five is also on its way to grabbing the transition and authentication area (e.g., payments, digital IDs).
Let’s take a look at CB Insight’s report on how exactly they are going to do that:
To start, WhatsApp users in certain countries can now search for businesses within the app, message them directly, and make payments in the chat. Meta is charting a course to handle discovery, messaging, and payments for WhatsApp’s more than 2B users globally.
Meanwhile, others are going after payments, a key enabler of super app functions. Apple Pay adoption has taken off in the US, with an estimated 75% of iPhones having the service activated. In May 2022, Google rolled out its digital wallet app for Android phones, which stores credit cards, transit documents, tickets, and more. Going a step further in owning the consumer journey end-to-end, Apple is reportedly looking to bring a variety of financial products in-house by developing its own tech for payment processing, fraud analysis, and more in a project dubbed “Breakout.” (source)
As a result, the traditional methods for discovering banking services have been disrupted, and most banks will need to now compete not only with other banking institutions but also with those not commonly recognized as financial services companies.
What should an AI-first bank be like?
- Intelligent = suggesting what to do, predicting important choices or tasks, and automating them.
- Personalized = using a good understanding of customers’ previous actions and current situation and offering relevant and timely solutions. AI-powered personalization significantly enhances user engagement and conversion rates. A McKinsey study found that companies excelling in personalization generate 40% more revenue than average players. In fact, intelligent apps & AI for personalization is one of the top AI trends for 2024.
- Extremely automated – automating manual tasks and decision-making.
- Fast and Agile – striving to be as fast and flexible as fintech apps (for example, by releasing updates every week instead of every month) while also handling the security measures and rules needed for traditional financial services companies.
- Omnichannel = banks will operate from a ‘jobs to be done’ perspective – focusing on what the user wants to achieve and facilitating it in every possible way.
This involves integrating digital and physical products and the core banking application with related nonbanking products.
The concept is aligned with the idea of seamless customer journeys across the web, mobile apps, call centers, and intelligent devices. Users can effortlessly transition between these touchpoints, all of which are updated and capable of promptly addressing their needs. For instance, if a user encounters an issue within the app, they can reach out to the call center, and the representatives are already aware of the problem they are facing. - EXTRA: Fun = there’s one less obvious AI-first bank feature that can make a difference: is it fun to use? You can make your app super-convenient with automation and personalization, but consider implementing gamification features to make users stick with your app for longer.
Applications of artificial intelligence (AI) in the banking industry
AI in banking goes beyond customer service to revolutionize everything, from front-end interactions to back-office automation. Let’s go through a few examples.
Security
Biometrics: Smile-to-pay & micro-expression recognition for fraud detection
AI biometrics refers to the application of artificial intelligence techniques to analyze and interpret individuals’ unique physical and behavioral traits (such as fingerprints, facial features, iris patterns, voiceprints, and even typing patterns) for authentication, identification, and security purposes.
In 2018, smile-to-pay and micro-expression recognition were two commonly commented artificial intelligence applications in China.
Smile-to-pay: customers can effortlessly complete purchases by standing in front of point-of-sale (POS) machines. These machines have cameras linked to a digital payment system or bank account through a facial image. (source)
Micro-expression: Chinese financial institutions have implemented micro-expression technology to detect potential fraud by analyzing subtle facial movements through smartphone cameras, aiming to identify customers who might be providing inaccurate information regarding loan purposes. (source)
Despite many followers of biometrics, serious ethical questions were raised, the main issue being: how are banks storing customer data, and what do they do with it?
Machine learning techniques for fraud detection and to support underwriting and risk management
Financial crimes can take various forms, including fraudulent credit card transactions, forged bank checks, tax evasion, money laundering, cyber attacks, customer account theft, synthetic identities, fake applications, and scams.
Fraud detection is a set of proactive measures undertaken to identify and prevent fraudulent activities and financial losses. Its main analytical techniques can be divided into two groups:
- Statistical: statistical parameter calculation, regression, probability distributions, data matching
- Artificial intelligence (AI): data mining, machine learning, deep learning
Machine learning represents an essential pillar for fraud detection. (source)
Automation
Optical character recognition to scan and process documents
Optical character recognition (OCR) has many applications, including document scanning. We’ve already adopted the solution for some of our clients or internal use (see: Health Folder: Your AI-based digital medical documentation folder and Saving 2 hours daily: efficient AI & low-code accounting automation).
These computer vision systems can check real papers before allowing changes to addresses. They could also be used to, for example, scan paper invoices and forms to save essential details about customers and transactions.
Virtual assistants for customer service
Banks can employ NLP (natural language processing)-backed chatbots to enhance customer engagement by enabling automated and conversational interactions for various banking-related tasks and inquiries.
Here’s an example of two leading financial institutions using chatbots:
HDFC Bank, an Indian bank, owns the first and largest AI-powered banking chatbot in India named Eva. This bot handles over 300,000 queries daily and has already responded to over 5 million queries from around a million customers with an accuracy rate of over 85%. Eva responds within fractions of a second. Similarly, SEB, a Swedish commercial bank, has created two chatbots: Aida for customer service and Amelia for internal IT communication among its 15,000 employees. Amelia receives around 300 different queries daily. (translation; source)
Robotic process automation for structured operational tasks
Bank staff manages large volumes of customer data, and relying on manual procedures can result in errors. Combining extensive data extraction and manual processing in banking operations can contribute to inaccuracies.
Robotic Process Automation (RPA) is a tech that uses software bots to automate repetitive tasks in business processes, like data entry, extraction, calculations, account opening, KYC processes, and more, typically done manually.
These software robots can perform structured tasks with high accuracy and speed, significantly reducing the likelihood of human errors. RPA increases operational efficiency and enables banks to allocate human resources to more strategic and value-added activities.
Personalization & intelligence
Recommender systems, another subset of AI technologies, have found valuable applications in the banking sector.
These systems help banks provide personalized and relevant recommendations to their customers, enhancing their financial experiences. They can, for example, provide banking customers with analytics-backed personalized offers or loan and credit decision recommendations.
What threats prevent banks from deploying AI technologies at scale?
The banking industry is one of today’s most highly regulated sectors. The implementation of AI introduces additional risks if not handled correctly.
According to McKinsey, the top three challenges that stop banks from using AI technologies extensively are a lack of clear strategy for AI, weak core technology and data backbone, and an outmoded operating model and talent strategy (source).
Apart from that, other issues impede the scaling of AI models in banks, such as handling customer information, ensuring high-quality, accurate, and relevant data, overcoming the bias of AI algorithms, and providing transparency of the AI models, particularly deep learning algorithms used by banking institutions.
Bottom line
Artificial intelligence has made its way into the finance sector, and seems it will be around for a while.
Banks must embrace AI-based systems to fuel innovation, improve customer service, boost customer experience, and not get out-competed by other financial services firms.
How Pragmatic Coders can help your bank become AI-first
With our extensive experience in fintech, the banking industry specifically, we can help you create your AI-first banking app.
Read the case study of Atom Bank, the UK’s first fully digital bank, or check other fintech case studies to learn more about our expertise in this area. If you have any questions regarding partnering with us, don’t hesitate to reach out to us: