Edge AI: Driving next-gen AI applications in 2024
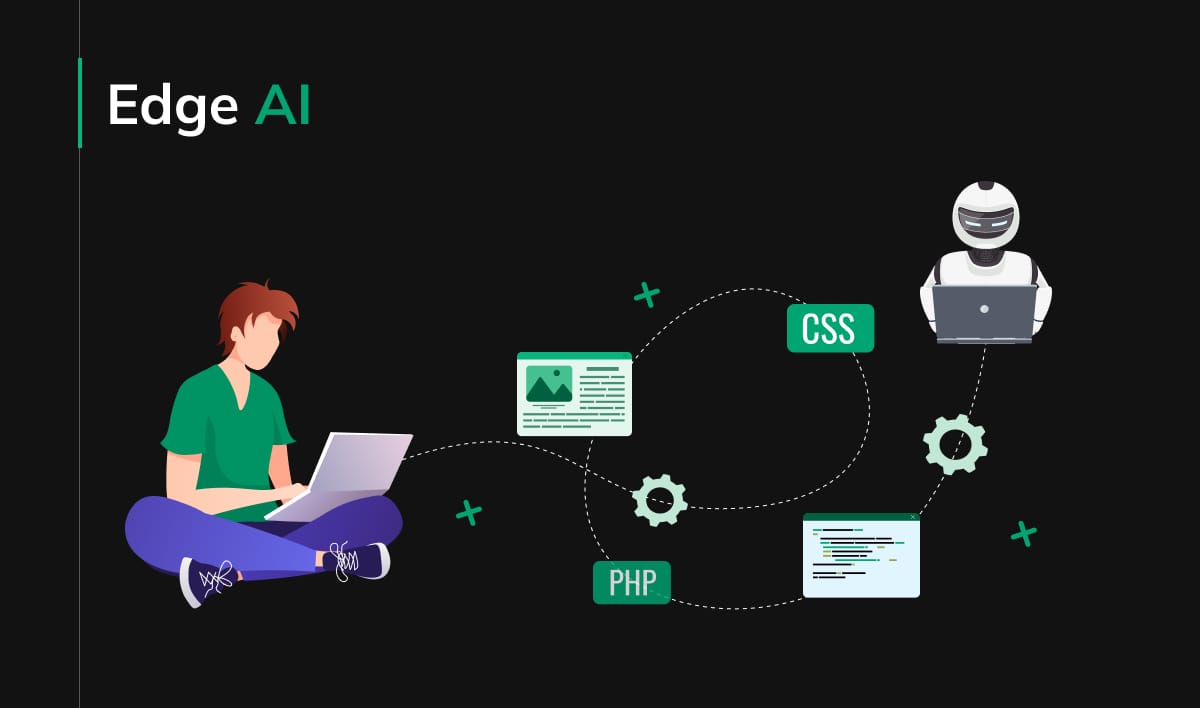
In 2021, the market size of edge AI was 11.98 billion and is projected to grow 107.47 billion by 2029. This shows a planned rise of 31.7 percent Compound Annual Growth Rate, i.e., CAGR. You might be wondering what is pushing this growth significantly. Rest assured, we are here to explain all about it, making it easy to proceed further.
Edge AI combines edge computing with AI to run AI and machine learning models directly on edge devices at the network edge instead of in the cloud.
This convergence of edge computing and AI is giving rise to next-generation applications. This is actioned to overcome the limitations of traditional cloud-based AI, such as increased latency, high bandwidth costs, and privacy and security concerns associated with moving large volumes of data to central cloud servers.
Edge AI enables real-time processing of data locally with minimal latency for applications that require instant decision-making or response.
Here, we are going to discuss how edge AI is shaping the future by driving the next wave of innovative AI applications across various industries in 2024 and beyond. Let’s get started.
Edge AI: What makes it a great asset?
Edge AI performs artificial intelligence and machine learning-related tasks at the edge of the network. To understand Edge AI in depth, a first understanding of AI Edge Computing individually and how they complement each other is important.
Artificial Intelligence (AI)
This refers to the machines’ proficiency to think and act intelligently like humans, namely learning new abilities, engrossing problem-solving skills, being able to reason, and many more.
Edge Computing
It refers to processing data and workload at the edge of the network, near the data sources, instead of sending large amounts of data to a centralized cloud data center. This helps in addressing issues like high latency, bandwidth constraints, and security concerns involved in sending everything to the cloud.
Edge AI
It involves performing AI-related tasks, like machine learning model training, directly on edge devices located near data sources like IoT sensors, cameras, etc. or on edge servers. This technology combines the of edge computing, like low latency and data sovereignty, with AI capabilities.
Key aspects that define Edge AI
Each platform supports processing data locally, on edge devices, and making intelligent decisions without sending raw data to the cloud. In fact, it plays a crucial role in deploying machine learning and deep learning for real-time inference.
Using the respective platform, you can also train and update models locally, on edge devices or edge servers, by making them learn from continuous data streams. It also helps in the process of managing and orchestrating a large network of edge devices and servers located at the network edge.
This shows how Edge AI plays an essential role; however, a question arises as to why it is needed. We have your answer crafted below :
Benefits of Edge AI
Thanks to Edge AI, devices can analyze data locally, leading to faster results, lower bandwidth use, and even greater privacy. Some key benefits of deploying AI capabilities at the network edge using Edge AI include:
Greater privacy and security
Sensitive user or device data is processed locally, in an edge computing environment, instead of being sent to public clouds.
Reduced latency
Edge AI enables real-time and low-latency processing. It is critical for applications like autonomous vehicles, augmented reality, etc.
Mobility support
Edge AI can work even in intermittent or no internet conditions.
Lower bandwidth usage
Only analyzed metadata is sent to the cloud instead of huge raw data volumes.
Cost savings
Lower costs on infrastructure and bandwidth compared to cloud-based approaches.
Location awareness
Models are trained based on localized patterns and behaviors for improved personalization and contextual awareness.
Physical architecture of Edge AI
Let’s understand the typical physical architecture of an Edge AI setup.
IoT or Edge devices
These are the endpoint devices like cameras, sensors, smartphones, etc. that collect and generate data.
Edge nodes
These are the processing units placed near the data sources, which can be edge servers, gateways, or even high-end smartphones or tablets capable of performing AI and ML tasks.
Edge network
Edge nodes are interconnected through network switches and routers to form an edge network. This can be either cabled or wireless.
Edge server
Powerful edge servers installed at network edges like local shops or offices can aggregate data from many edge nodes and perform advanced ML tasks.
Core network
Edge servers are connected to the core network that transports data between edges and clouds.
Cloud
Hyperscale cloud data centers that provide higher computation, longer-term storage, and centralized ML model management capabilities.
Back-end apps
It is an enterprise application consuming insights from edge-based AI and ML models.
Edge AI platforms
Managing this distributed edge-cloud physical architecture requires specialized edge AI software platforms that can register, authenticate and manage a huge network of edge devices and servers.
It must also be able to push AI models to edges, update models securely over-the-air, and orchestrate model training across the edge-cloud continuum. The platform must also be able to collect, process, analyze, and visualize edge data and trigger automations based on real-time edge insights. It also ensures the security, reliability, and scalability of edge operations.
Some leading commercial Edge AI platforms include:
AWS IoT Greengrass
It is an edge solution from AWS to build, deploy, and manage device software.
Azure IoT Edge
It is Microsoft’s IoT edge computing platform, used to deploy containerized edge apps.
IBM Watson IoT Platform
This platform enables connecting, managing, and analyzing IoT data at the edge.
Anthropic Fleet
It is used by a full-stack, scalable platform to deploy and monitor AI and ML models on the edge.
Viso.AI
It provides a unified platform to build, deploy, and manage computer vision applications across the edge cloud.
C3 IoT
It is an end-to-end IoT platform, focusing on industrial applications with edge capabilities.
Octonion
It is a platform utilized for distributing data and providing low-latency deep learning across the edge, core, and cloud.
Multi-Access Edge Computing (MEC)
Multi-Access Edge Computing, i.e., MEC, refers to an edge computing architecture standardized by the European Telecommunications Standards Institute, i.e., ETSI, to support 5G networks. In MEC, the traditional cellular base stations are augmented with edge servers to enable low-latency mobile edge applications.
MEC servers are placed at Radio Access Network, i.e., RAN, nodes near end users for ultra-low latency. It integrates tightly with 5G cellular infrastructure for enhanced mobile broadband services.
It supports edge application deployment and enhances services for better user experience in areas of AR and VR, IoT, autonomous vehicles, etc. In fact, it also leverages live geolocation detection through cloud computing capabilities and radio network intelligence.
This shows how mobile edge computing plays a critical role in enabling Edge AI capabilities for 5G-enabled use cases like autonomous driving, remote healthcare, smart manufacturing, etc. MEC addresses the needs for real-time, location-aware edge processing driven by 5G networks and use cases.
Edge AI Use cases
With its ability to overcome the latency and privacy challenges of the cloud, it is expected to drive numerous artificial intelligence applications across various industries in 2024 and beyond.
Smart manufacturing
Intelligent systems powered by Edge AI will monitor equipment health, optimize production processes, ensure quality and coordinate logistics in real-time on factory floors for predictive maintenance and flexibility.
Autonomous vehicles
Edge AI will power autonomous driving features by enabling vehicles to process large volumes of data from cameras, radar and LiDAR sensors in real-time for critical functions like lane departure warning, automatic emergency braking, traffic sign recognition, etc.
Augmented and virtual Reality
Edge servers will process intensive computer vision and sensor data to enable real-time object detection, tracking, and rendering of 3D virtual environments on resource-constrained AR and VR headsets.
Remote healthcare
Edge AI-based systems will monitor patients remotely, enable AI radiology and diagnostics at rural clinics, and provide power-assisted tele-rehabilitation therapy through real-time analysis of bio-sensor data.
Smart cities
Edge devices, like smart traffic cameras and environmental sensors, will use AI to optimize traffic management, monitor air quality, and manage energy and water distribution to ensure public safety with low-latency insights.
Education
Adaptive learning platforms deliver hyper-personalized education by profiling each student’s learning patterns using real-time analysis of in-class sensors powered by Edge AI.
Live events and entertainment
Live crowds, stadium or venue monitoring, and visitors’ flow optimization using Edge AI-based computer vision and behavioral analytics to enhance security and mass management.
Retail and supply chains
Intelligent retail assistants and computer vision-based inventory tracking solutions deployed at retail stores or warehouses will optimize customer experience, shelf management, and logistics using Edge AI.
AI at the edge. Final thoughts
As more and more devices are getting connected and generating massive amounts of data, there is a need to process this data in real-time and make intelligent decisions. This is where Edge AI comes into the picture.
With advancements in technologies like the Internet of Things (IoT), 5G, edge computing, and AI algorithms, we are witnessing a digital transformation across various industries.
It shall be inevitable if it is said that no lives will remain untouched by the influence of Edge AI. It carries the mighty power to improve climatic conditions, bring great enhancements to health, decrease road rage, and do anything one can imagine.